The Role of AI in Candidate Review: Balancing Efficiency with Fairness in Hiring
Updated: Tue, Oct 29, 2024
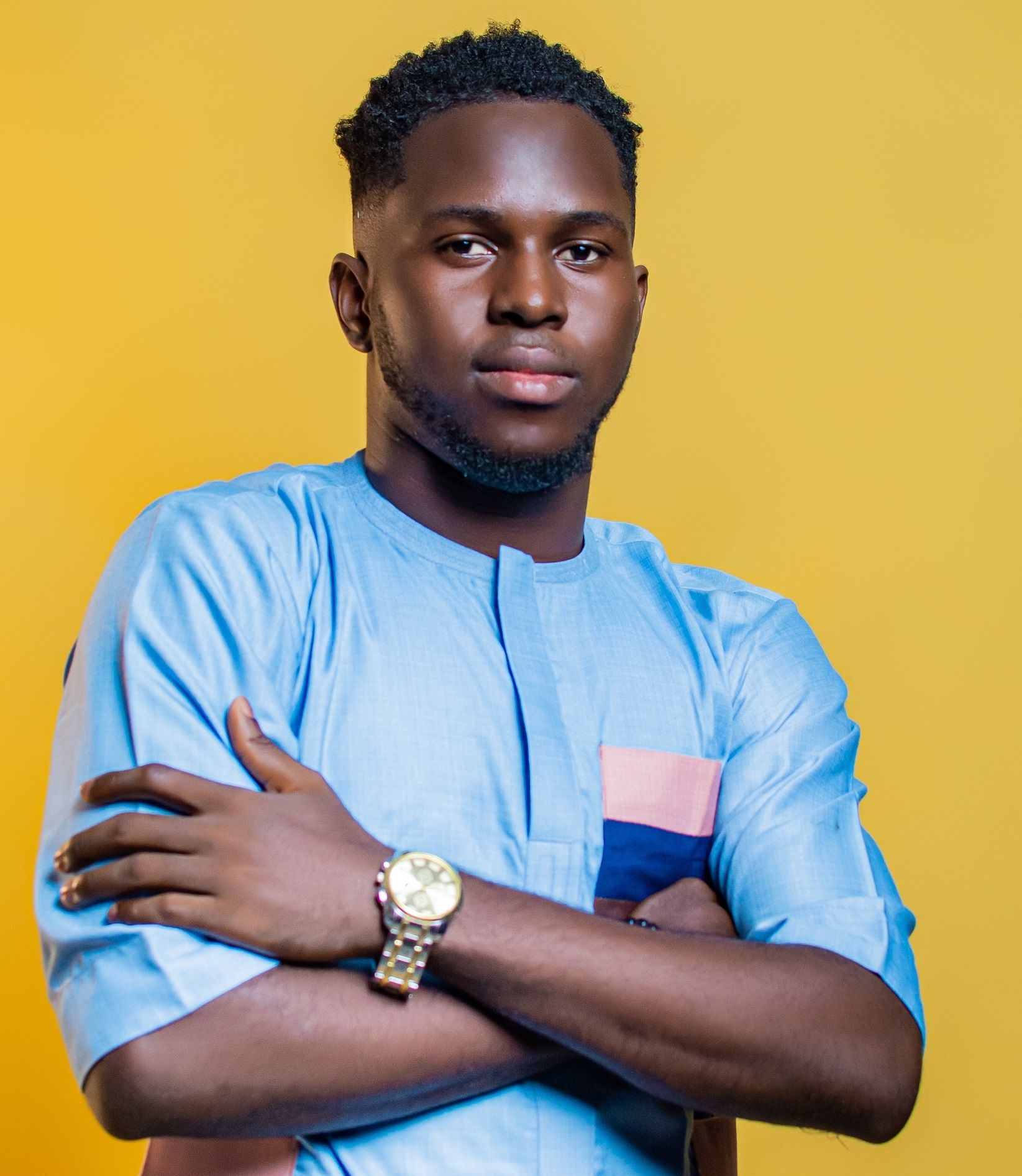
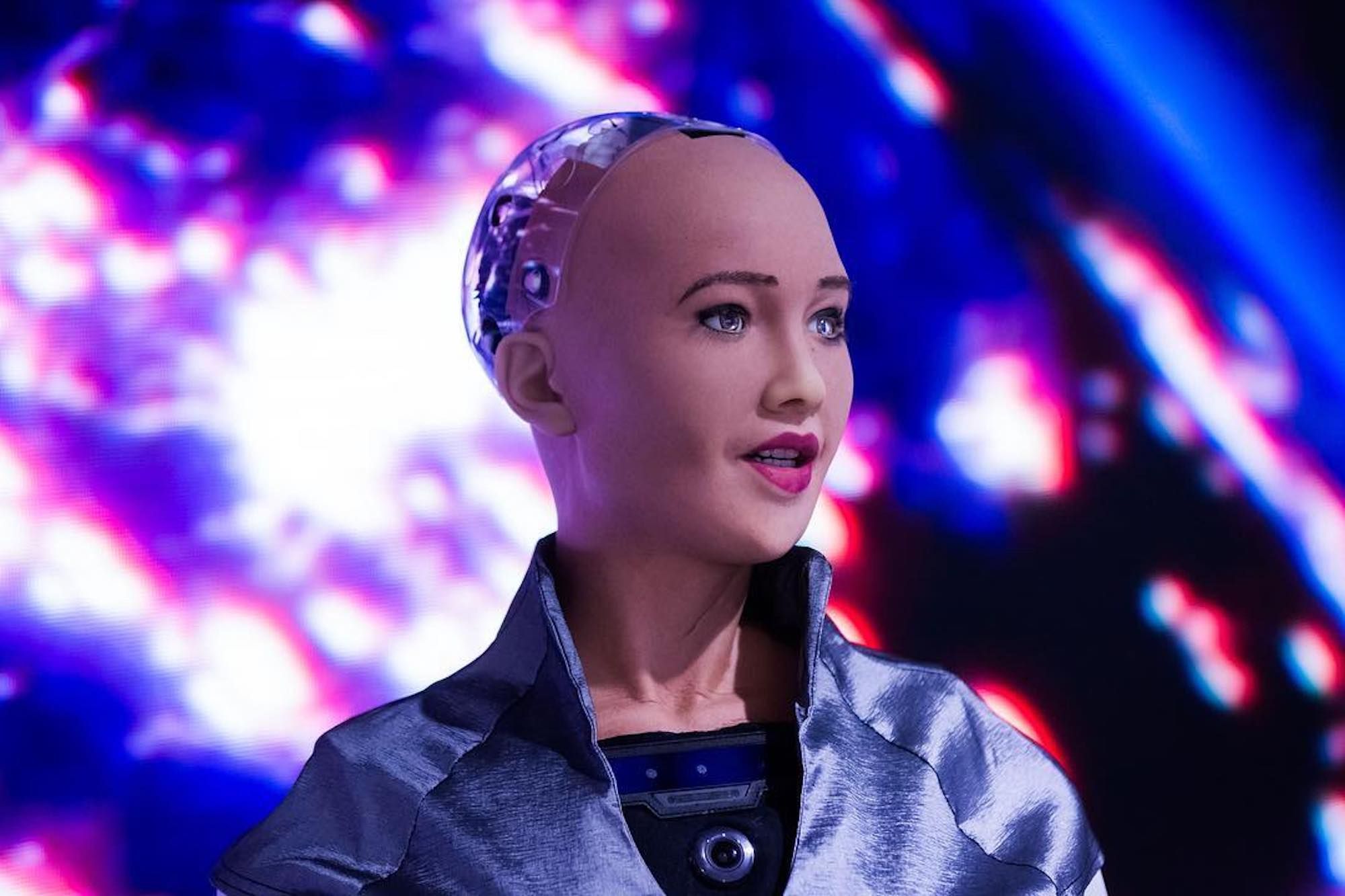
The integration of AI in candidate review processes is transforming talent evaluation in hiring processes today. This is because AI tools can simplify and optimize the recruitment process, sifting through resumes and identifying top candidates in a fraction of the time it used to take. While efficiency is essential, we can’t overlook the critical aspect of fairness in hiring decisions.
As organizations increasingly rely on AI, it’s vital to strike a balance between speed and equity. I believe understanding the potential biases in AI algorithms is crucial for creating a more inclusive hiring environment. In this article, I’ll explore how AI can enhance candidate review while ensuring fairness remains at the forefront of the recruitment process.
Understanding AI in Recruitment
AI in recruitment encompasses automated systems and algorithms that analyze candidate data, optimize workflows, and support hiring decisions. AI tools process large volumes of resumes, assess qualifications, and match candidates to job roles based on defined criteria. Through utilizing machine learning and natural language processing, AI systems draw insights from behavioral and demographic data. These insights facilitate quick identification of top candidates while aiming to reduce human error and bias in evaluations.
Common AI Tools Used in Candidate Review
Several AI tools enhance the candidate review process. Here’s a list of prominent tools:
- Applicant Tracking Systems (ATS): ATS automates the collection and organization of resumes, enabling recruiters to filter candidates based on specific keywords or qualifications.
- Resume Screening Software: These tools use algorithms to analyze keywords and experience, allowing organizations to prioritize resumes that closely match job descriptions.
- Chatbots: Chatbots engage with candidates during the application process, answering questions and gathering initial information, enhancing candidate experience while saving time for recruiters.
- Predictive Analytics Tools: These tools assess historical hiring data to predict candidate success and identify traits linked to high-performing employees in specific roles.
- Bias Detection Tools: Such tools analyze recruitment processes to identify and mitigate bias, helping organizations foster a more inclusive hiring environment.
- Video Interviewing Platforms: These platforms utilize AI to assess candidate responses and behaviors during interviews, providing additional layers of insight for recruiters.
Incorporating these AI tools, organizations can enhance their candidate review processes, balancing efficiency with fairness in hiring practices.
The Role of AI in Candidate Review: Balancing Efficiency and Fairness
AI has transformed the candidate review process by automating time-consuming tasks and improving efficiency. From scanning resumes for keywords to analyzing candidates' qualifications based on preset parameters, AI enables recruiters to make faster, data-driven decisions. However, the rapid adoption of AI in hiring also brings complex challenges, particularly around fairness and bias. By addressing these challenges and combining AI capabilities with human insight, organizations can leverage AI as a tool for equitable, effective hiring.
Enhancing Efficiency in Candidate Review
One of AI's primary advantages in recruitment is its ability to streamline the candidate review process. Advanced algorithms can analyze thousands of applications within minutes, highlighting applicants whose experience and skills best match the job criteria. This reduces the time recruiters spend on initial candidate screenings and allows them to focus on deeper evaluations of the top candidates.
- Automated Resume Parsing: AI-driven systems can parse resumes, identify qualifications, and rank candidates based on specific skills, experience levels, and educational backgrounds. This ensures that key qualifications aren’t missed, particularly for high-volume roles with hundreds or even thousands of applicants.
- Predictive Analytics: Many AI tools can predict a candidate’s likelihood of success based on past hiring data, offering insights into potential cultural fit or performance expectations. Predictive analytics models help hiring teams prioritize candidates whose profiles align well with organizational needs.
Automating routine tasks, AI enables faster, more consistent candidate evaluations and reduces the risk of human error. However, speed and efficiency alone do not guarantee a fair process, which is where the ethical considerations of AI in hiring become crucial.
Addressing Fairness and Mitigating Bias
AI is only as unbiased as the data it is trained on, and recruitment algorithms are vulnerable to inheriting biases from historical hiring data. For instance, if a company’s past hiring practices unintentionally favored certain demographics, an AI model trained on this data could reproduce those biases, potentially excluding qualified candidates from underrepresented groups. To counteract these risks, organizations must approach AI-driven hiring with a commitment to fairness and rigor.
- Bias Detection and Regular Audits: One way to address potential AI biases is through frequent model evaluations. By regularly auditing the algorithms and reviewing outcomes, hiring teams can spot patterns of potential discrimination, such as favoring or excluding candidates based on gender, ethnicity, or educational background. This proactive approach allows organizations to adjust criteria, retrain models, and refine algorithms to reduce bias.
- Inclusive Training Data: Another essential factor in fairness is ensuring that AI models are trained on diverse, representative datasets. Including data from various demographics and backgrounds, companies can improve the inclusivity of AI-driven candidate reviews. Collaboration with diverse groups during the design and testing phases ensures multiple perspectives are considered, helping to mitigate bias from the outset.
Transparency and Accountability in AI Decision-Making
To foster trust in AI-driven hiring, organizations should emphasize transparency in AI decision-making processes. When candidates and hiring teams understand how AI selects and ranks applicants, they’re more likely to trust the outcomes and feel confident that the process is fair.
- Clear Communication of AI’s Role: Companies can build candidate trust by explaining how AI tools are used in the hiring process. Candidates should know whether AI is involved in resume screening, assessment scoring, or initial ranking and how it influences hiring decisions. This clarity helps candidates see AI as a fair tool rather than a mysterious “black box” that makes decisions without accountability.
- Human Oversight: AI in hiring works best as an assistive tool, not as a final decision-maker. It's good practice to ensure human oversight at every stage of the process since organizations allow recruiters to apply their own judgment, intuition, and understanding of nuanced candidate qualities that AI might miss. For example, recruiters can consider factors like soft skills and cultural alignment that aren’t easily quantified by AI.
Get instant hire/no-hire recommendations about shortlisted candidates
Use AI-powered scorecards to collect and analyze feedback your candidates will thank you for
A Collaborative Approach: Integrating AI Efficiency with Human Insight
AI brings remarkable efficiency to candidate review, but human judgment remains essential for balanced, thoughtful hiring decisions. Recruiters can leverage AI as a valuable aid, using its data processing power to complement their expertise and insight.
- Enhanced Candidate Screening: AI can handle the repetitive aspects of screening, but human recruiters add value by evaluating a candidate's personality, communication style, and potential for growth. This collaboration allows teams to focus on building relationships with candidates, fostering a more personal and respectful hiring process.
- Data-Driven Insights and Human Intuition: While AI excels at identifying patterns in past hiring data, recruiters bring unique intuition and empathy to the process. For instance, a candidate with unconventional experience might be overlooked by an algorithm, but a recruiter could recognize the potential value of their diverse background. This combination of data-driven insights and human intuition ensures that hiring decisions account for both skills and cultural fit.
- Feedback Loop for Continuous Improvement: A collaborative AI-human approach creates a feedback loop where recruiters review AI-generated recommendations and provide input to improve the model over time. This dynamic adjustment not only increases the accuracy of candidate reviews but also ensures that the AI model remains aligned with the company’s evolving values and needs.
Table of Contents
Featured Podcast
Improve candidate experience in 7 minutes. Listen now.
FAQs
More information about this topic